Unveiling the Core Concepts of Unsupervised Learning in Generative AI
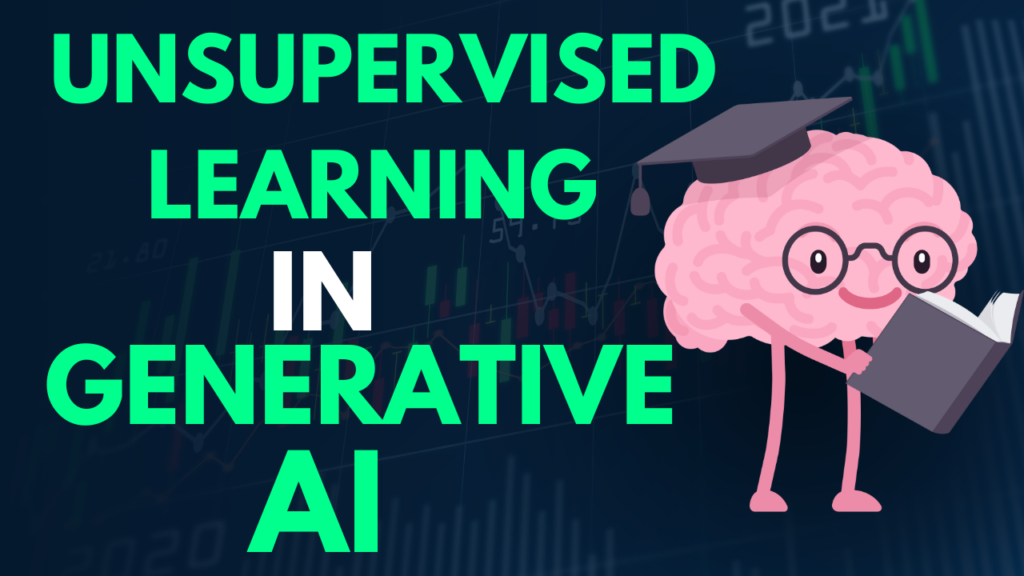
Unsupervised learning lies at the heart of generative AI, allowing machines to learn from unlabelled data and discover patterns independently. In the context of generative models, unsupervised learning involves training models to generate new data samples that resemble a given dataset. This process is particularly valuable when labeled data is scarce or expensive to obtain. By unraveling the core concepts of unsupervised learning in generative AI, we delve into the mechanisms that drive machines to understand the underlying structures of data, enabling them to create new content that reflects the essence of the original dataset.
Diving into Generative Models: How Unsupervised Learning Drives AI Creativity
Generative models are the creative powerhouses of AI, and unsupervised learning is the driving force behind their innovation. By training these models on unlabelled data, they gain the ability to generate new content that captures the intricacies of the training dataset. This article takes a deep dive into the world of generative models, exploring how unsupervised learning fuels AI creativity. We explore the artistic potential of these models, their role in diverse industries, and the magic that happens when machines learn to create without explicit instructions.
Gaining Insights: The Role of Latent Representations in Unsupervised Learning
At the heart of unsupervised learning in generative AI lies the concept of latent representations. These are compact, high-dimensional vectors that capture the essence of data. In generative models, latent representations hold the key to generating meaningful content. This article delves into the pivotal role of latent representations in unsupervised learning, discussing how these vectors encode the essential features of data and enable the generation of new, coherent content. By understanding latent representations, we unlock the gateway to AI’s ability to produce realistic and imaginative outputs.
Autoencoders: Building Blocks of Unsupervised Learning in Generative AI
Autoencoders are foundational in unsupervised learning for generative AI. These neural networks encode input data into a compressed representation and then decode it to reconstruct the original input. In the realm of generative models, autoencoders serve as essential building blocks for creating diverse content. This article explores the mechanics of autoencoders, how they facilitate feature extraction, and their role in generating novel outputs that resonate with the characteristics of the input data.
Generative Adversarial Networks (GANs): Redefining Unsupervised Learning
Generative Adversarial Networks, or GANs, have redefined the landscape of unsupervised learning. GANs consist of two neural networks, a generator, and a discriminator, engaged in a creative duel. This article delves into how GANs revolutionize unsupervised learning by setting these networks against each other to generate content that is increasingly realistic. By understanding the intricate dance of GANs, we uncover the innovative technique that has enabled machines to produce astonishingly convincing outputs.
Variational Autoencoders (VAEs): Unleashing Creative Potential through Unsupervised Learning
Variational Autoencoders, or VAEs, empower generative models to unleash their creative potential. Unlike traditional autoencoders, VAEs model data using probability distributions, enabling the generation of diverse outputs. This article explores how VAEs leverage unsupervised learning to capture the underlying structure of data and use it to synthesize novel content. By understanding VAEs, we gain insight into how these models elevate generative AI to produce content that spans a spectrum of possibilities.
Unsupervised Learning vs. Supervised Learning: A Closer Look at Generative AI
Unsupervised learning and supervised learning are two pillars of machine learning, each with distinct purposes. In the realm of generative AI, unsupervised learning shines as it enables models to generate content without explicit labels. This article offers a comparative analysis of unsupervised learning and supervised learning in the context of generative models. By delving into their differences and benefits, we unravel why unsupervised learning is the driving force behind the creative capabilities of AI.
Applications of Unsupervised Learning in Generative Image Synthesis
Unsupervised learning’s impact on generative image synthesis is profound and far-reaching. By learning patterns from unlabelled data, generative models can craft realistic and diverse images. This article delves into the applications of unsupervised learning in generative image synthesis. From art creation to medical imaging, from data augmentation to content generation, we explore how unsupervised learning is reshaping industries and fueling the development of AI-driven creative solutions.
Text Generation Unleashed: Unsupervised Learning in Language Models
The art of text generation is revolutionized by unsupervised learning in language models. By analyzing vast amounts of unlabelled text data, language models learn the intricacies of language and generate coherent, contextually relevant text. This article unveils how unsupervised learning drives language models, enabling them to produce captivating narratives, creative writing, and even chatbot interactions that mimic human communication. Dive into the world of text generation, where machines learn to express themselves through words.
Future Horizons: Innovations and Trends in Unsupervised Learning for Generative AI
As technology evolves, the future of unsupervised learning in generative AI holds exciting potential. This article peers into the future, exploring the innovations and trends that will shape this dynamic field. From multi-modal learning to unsupervised reinforcement learning, from interpretability to ethical considerations, we delve into the advancements that are set to redefine the landscape of AI-driven creativity. By understanding these trends, we prepare for a future where unsupervised learning takes generative AI to unprecedented heights.
Conclusion
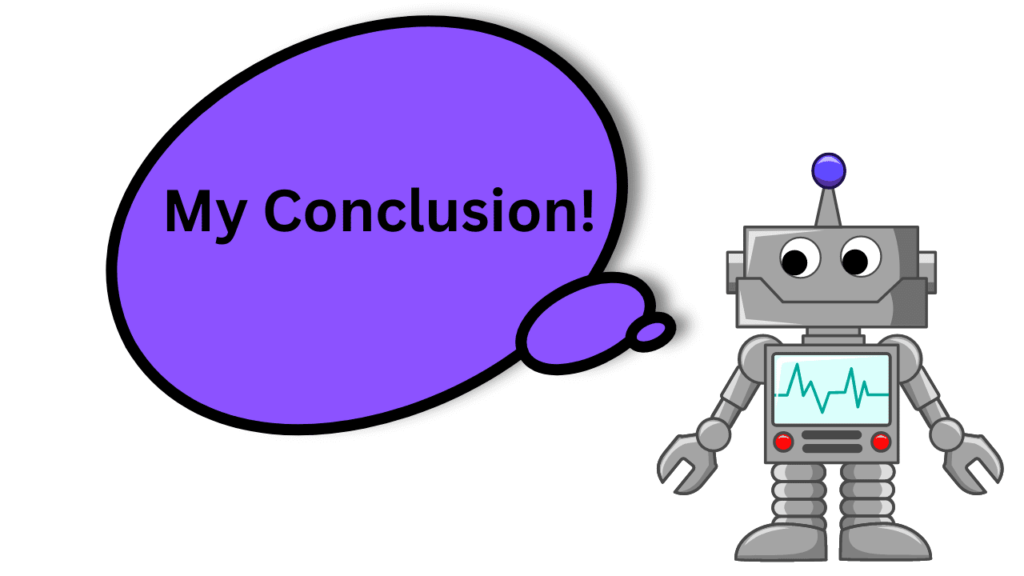
The journey into the world of unsupervised learning in generative AI is a mesmerizing exploration of how machines attain creativity. By delving into the core concepts, diving into generative models, and understanding the role of latent representations, we’ve glimpsed the magic that underpins AI’s ability to create from scratch. Autoencoders, GANs, and VAEs serve as the cornerstones, driving machines to generate content that ranges from realistic to imaginative.
Comparing unsupervised and supervised learning, we recognize that unsupervised learning is the secret ingredient that fuels the AI’s creative fire, allowing it to craft without constraints. Its applications span from image synthesis to text generation, demonstrating its impact across industries. With an eye towards the future, we foresee trends and innovations that will redefine the boundaries of generative AI.
As we conclude this journey, we find ourselves at the threshold of a new era, where AI-generated creativity stands as a testament to human ingenuity and innovation. Unsupervised learning is the vehicle that carries us into this future, a future where machines paint, write, and compose with a touch of artistic brilliance. With each advancement, each stroke of generated brilliance, we see a world where AI and creativity are woven together in an intricate dance of innovation.