Introduction to Deep Reinforcement Learning: Basics and Concepts
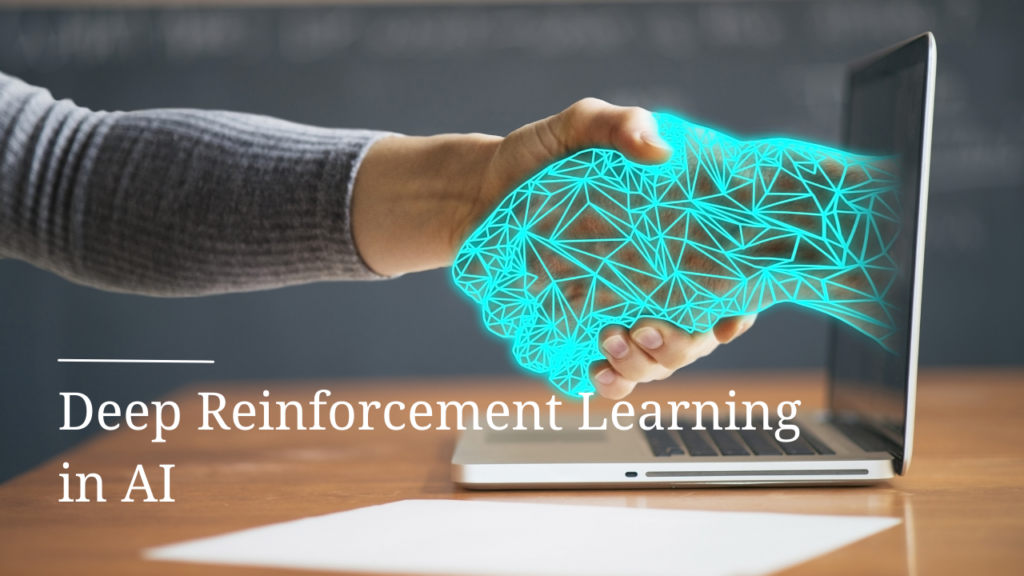
In the dynamic realm of artificial intelligence, Deep Reinforcement Learning (DRL) stands as a powerful paradigm that fuses the principles of reinforcement learning with the capabilities of deep neural networks. At its core, DRL involves training agents to make sequential decisions in an environment, aiming to maximize cumulative rewards over time. This synergy enables machines to learn and adapt through interaction, paving the way for applications in gaming, robotics, finance, healthcare, and more. Introduction to Deep Reinforcement Learning: Basics and Concepts lays the foundation for understanding this cutting-edge field, exploring the fundamental terminology, concepts, and mechanisms that underpin its operation.
Key Algorithms in Deep Reinforcement Learning Explained
Within the intricate tapestry of Deep Reinforcement Learning (DRL) lie key algorithms that drive its advancements. From the cornerstone Q-learning algorithm, which forms the basis of value-based methods, to the nuanced Policy Gradient methods that optimize agent policies directly, each algorithm contributes unique insights to decision-making in DRL. Dive into an exploration of the Key Algorithms in Deep Reinforcement Learning Explained to unravel the mechanics and significance of Q-learning, DDPG, A3C, and more, and discover how they shape the capabilities of DRL agents.
Applications of Deep Reinforcement Learning in Real-World Scenarios
Applications of Deep Reinforcement Learning (DRL) extend far beyond theoretical constructs, permeating real-world industries and domains. From autonomous vehicles navigating complex urban environments to medical systems optimizing treatment strategies, DRL empowers machines to tackle intricate tasks with finesse. Uncover the versatility and impact of DRL in practical scenarios through the lens of Applications of Deep Reinforcement Learning in Real-World Scenarios, as we explore how this technology redefines the boundaries of what machines can achieve.
Deep Q-Networks (DQN): Revolutionizing Reinforcement Learning
The advent of Deep Q-Networks (DQN) marks a pivotal moment in the realm of Reinforcement Learning (RL). By coupling Q-learning with deep neural networks, DQNs bring learning from raw sensory input to the forefront. This groundbreaking approach has revolutionized agent training, enabling them to learn directly from pixel-level data. Delve into the mechanics of DQNs and their implications for the future of Reinforcement Learning, as we uncover how this innovation is redefining the landscape of intelligent decision-making in Deep Q-Networks (DQN): Revolutionizing Reinforcement Learning.
Policy Gradient Methods: Enhancing Deep Reinforcement Learning
At the forefront of Deep Reinforcement Learning (DRL) lies the power of Policy Gradient Methods. These techniques offer a distinctive approach to training agents by directly optimizing their policy functions. Unlike value-based methods, Policy Gradient Methods excel in scenarios that demand nuanced, continuous action spaces. Explore the intricacies of these methods and witness their role in enhancing the capabilities of DRL agents in Policy Gradient Methods: Enhancing Deep Reinforcement Learning.
Solving Complex Games with Deep Reinforcement Learning Techniques
Solving Complex Games has been a hallmark achievement of Deep Reinforcement Learning (DRL) techniques. From conquering board games like Go and chess to dominating video games with human-level performance, DRL techniques showcase the potential of intelligent decision-making in competitive scenarios. Delve into the realm of Solving Complex Games with Deep Reinforcement Learning Techniques to witness the triumphs and strategies that have propelled DRL to the forefront of strategic gameplay.
Challenges and Future Directions in Deep Reinforcement Learning
As the field of Deep Reinforcement Learning (DRL) matures, it faces an array of challenges that pave the way for future advancements. From the dilemma of sample inefficiency to addressing ethical concerns, these hurdles guide the trajectory of DRL research. Embark on a journey through the landscape of Challenges and Future Directions in Deep Reinforcement Learning, where we explore the obstacles researchers grapple with and envision the potential breakthroughs that await on the horizon.
Comparing Deep Reinforcement Learning and Traditional RL Approaches
The evolution of Deep Reinforcement Learning (DRL) has ushered in a new era of decision-making, challenging traditional Reinforcement Learning (RL) approaches. By contrasting the strengths and limitations of both paradigms, we gain a deeper understanding of when to employ each. Engage in a comprehensive exploration of Comparing Deep Reinforcement Learning and Traditional RL Approaches, where we dissect the nuances, trade-offs, and scenarios best suited for these distinctive methodologies.
Deep Reinforcement Learning in Autonomous Systems and Robotics
Deep Reinforcement Learning (DRL) has ignited a revolution in the field of Autonomous Systems and Robotics. By imbuing machines with the ability to learn and adapt, DRL empowers robots to navigate complex environments, make informed decisions, and optimize their actions. Explore the symbiotic relationship between DRL and autonomy as we delve into the applications, challenges, and potential of Deep Reinforcement Learning in Autonomous Systems and Robotics.
Ethical Considerations in the Development of Deep Reinforcement Learning
As the capabilities of Deep Reinforcement Learning (DRL) systems advance, so do the ethical considerations that surround their development and deployment. From algorithmic biases to accountability and transparency, the responsible use of DRL demands careful examination. Embark on a thought-provoking exploration of Ethical Considerations in the Development of Deep Reinforcement Learning, as we navigate the ethical dimensions of creating AI systems that align with societal values and ensure a just and equitable future.
Conclusion on Deep Reinforcement Learning
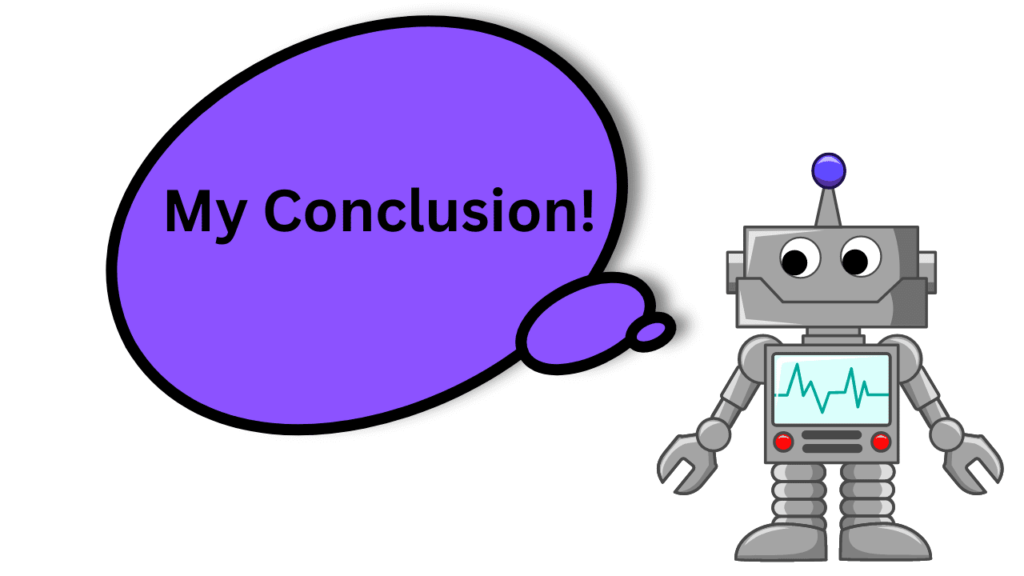
In conclusion, Deep Reinforcement Learning (DRL) stands as an innovative fusion of AI and decision-making, showcasing its prowess across diverse fields. With key algorithms like Q-learning and Policy Gradient Methods, DRL agents master complex scenarios. Applications span autonomous systems, robotics, gaming, and more. DRL’s transformative potential is evident in the revolutionary Deep Q-Networks (DQN), while ethical considerations guide responsible development. As DRL navigates challenges and paves future directions, comparing it with traditional approaches underscores its dynamism. The synergy of DRL in autonomous systems reshapes robotics. From basics to ethics, DRL redefines how machines learn and interact, shaping the landscape of intelligent technology.