1. Understanding the Fundamentals of Generative Modeling in AI
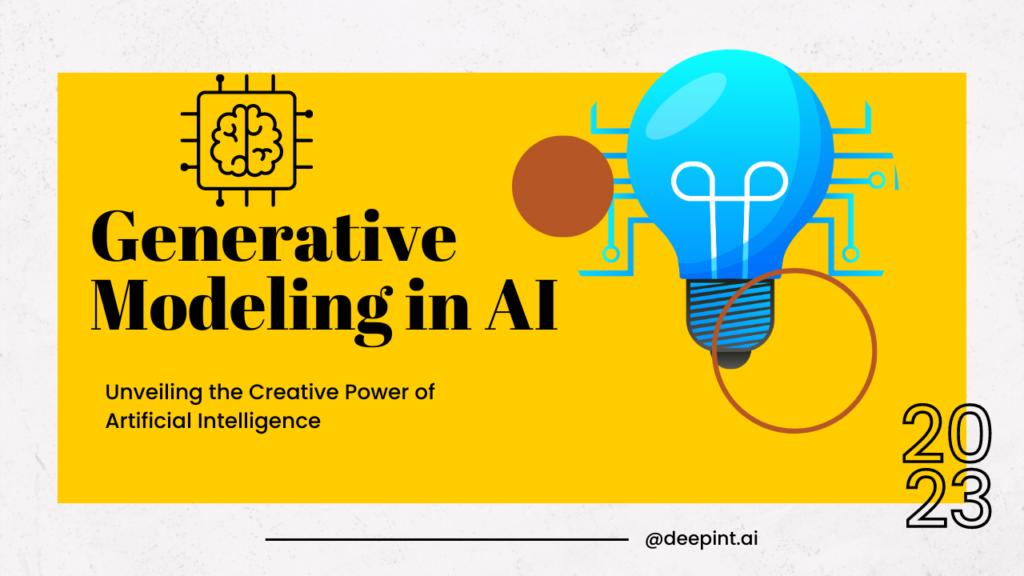
Generative modeling in AI is a foundational concept that empowers machines to create data resembling real examples from a given dataset. It involves training algorithms to learn patterns and relationships within data, enabling them to generate new content autonomously. By grasping the fundamentals of generative modeling, you gain insights into how AI can replicate and innovate upon existing information. This knowledge paves the way for applications in various fields, from art and design to healthcare and language processing.
2. Exploring Applications of Generative Modeling in AI
Generative modeling finds wide-ranging applications in AI-driven innovation. From generating lifelike images to creating realistic text, its potential knows no bounds. Businesses leverage generative models for content creation, while artists use them to produce novel artworks. Moreover, in healthcare, generative models assist in generating medical imagery and drug discovery. By exploring these applications, you unlock a world of possibilities where AI can transform industries through creative and data-driven output.
3. A Deep Dive into Variational Autoencoders for Generative AI
Variational Autoencoders (VAEs) stand at the forefront of generative AI techniques. They merge autoencoder architecture with probabilistic inference, allowing them to capture complex data distributions. VAEs learn latent representations of input data, enabling the generation of new instances that share similar traits. This deep dive into VAEs delves into their mathematical underpinnings, training processes, and showcases their proficiency in generating high-dimensional data, making them a cornerstone in the field of generative modeling.
4. Generative Adversarial Networks (GANs): Revolutionizing AI Creativity
Generative Adversarial Networks (GANs) have revolutionized AI creativity by introducing a two-part framework: a generator and a discriminator. These neural networks compete against each other, refining their outputs iteratively. GANs have demonstrated remarkable capabilities in producing photorealistic images, artistic creations, and even enhancing existing content. Understanding the mechanism of GANs sheds light on their potential to revolutionize content creation and manipulation.
5. Generating Realistic Images with Conditional GANs in AI
Conditional Generative Adversarial Networks (cGANs) take GANs a step further by incorporating additional information during the generation process. They enable the synthesis of images based on specific input conditions, leading to contextually relevant outputs. Whether it’s altering facial expressions or modifying scene attributes, cGANs offer a versatile approach to generating images that align with desired specifications. This topic explores the architecture and benefits of cGANs, showcasing how they excel in controlled image synthesis tasks.
6. The Role of Recurrent Neural Networks in Language Generation
Recurrent Neural Networks (RNNs) play a pivotal role in language generation tasks. Unlike traditional neural networks, RNNs possess memory, enabling them to process sequences of data. This attribute makes them invaluable in tasks such as text generation, machine translation, and speech recognition. By comprehending the role of RNNs in language generation, you unlock insights into the mechanisms behind coherent and contextually accurate text creation.
7. From Data to Art: How Neural Style Transfer Utilizes Generative AI
Neural Style Transfer (NST) exemplifies the fusion of art and technology through generative AI. By leveraging deep neural networks, NST transfers the artistic style of one image to the content of another, resulting in visually captivating amalgamations. Understanding the inner workings of NST allows you to appreciate the intricate balance between content and style representation in images, showcasing the potential of generative AI in artistic expression.
8. Enhancing Healthcare with Generative Modeling: A Case Study
Generative modeling isn’t confined to artistic domains alone; it’s also making strides in healthcare. This case study explores how generative models aid in medical image synthesis, enabling the generation of synthetic data for training without compromising patient privacy. By harnessing the power of generative models, healthcare professionals can enhance diagnostics, treatment planning, and medical research, illustrating the transformative impact of AI in healthcare.
9. Unsupervised Learning and Generative Modeling: A Symbiotic Relationship
Generative modeling and unsupervised learning share a symbiotic relationship that fuels AI advancements. Unsupervised learning empowers models to identify patterns in data without explicit labels, while generative models extend this concept by creating new data instances from these learned patterns. By delving into this relationship, you gain a profound understanding of how these techniques collaborate to drive AI’s ability to learn and create independently.
10. Ethical Implications of AI-Driven Generative Modeling
While AI-driven generative modeling offers remarkable capabilities, it also raises ethical considerations. This topic delves into the implications of using AI to create content, exploring issues such as intellectual property, deepfakes, and biases present in generated data. Understanding the ethical landscape surrounding generative AI is crucial for responsible development and usage, ensuring that technological advancements align with societal values and norms.
Conclusion
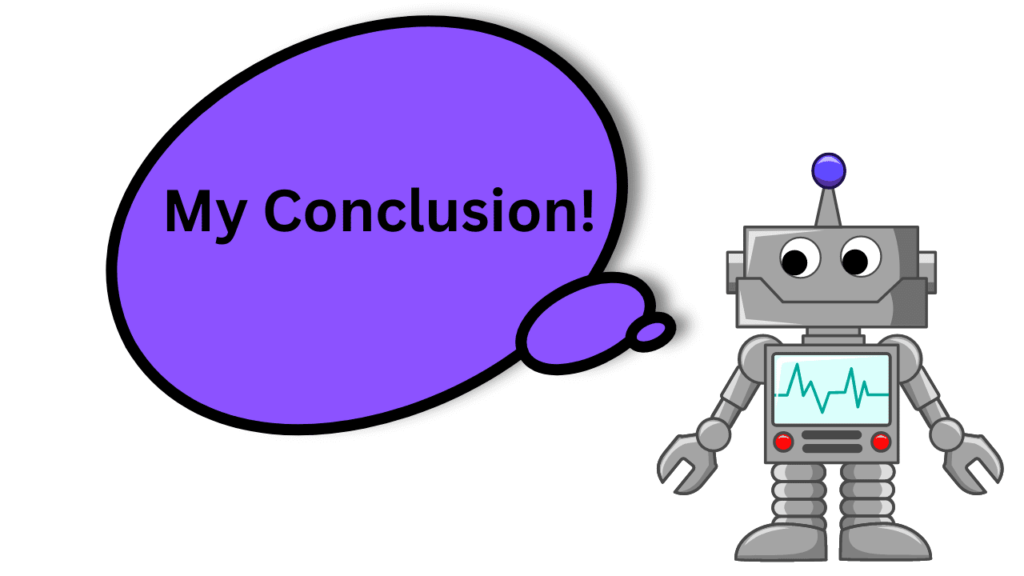
In conclusion, the realm of generative AI is a captivating landscape where innovation meets creativity, and technology intersects with imagination. From understanding the fundamental principles that underlie generative modeling to exploring its multifaceted applications across industries, the potential of AI to create, replicate, and enhance content is truly boundless. The journey through topics like Variational Autoencoders (VAEs), Generative Adversarial Networks (GANs), Recurrent Neural Networks (RNNs), and Conditional GANs showcases the intricate mechanisms behind data-driven creativity and problem-solving. As we delve into the synergistic relationship between unsupervised learning and generative modeling, it’s clear that these concepts intertwine to push the boundaries of AI’s capabilities. However, with great power comes responsibility, and an understanding of the ethical considerations surrounding AI-generated content is paramount. The fusion of technology, ethics, and imagination shapes the future of AI-driven generative modeling, where responsible development and creative exploration go hand in hand, driving us towards a more innovative and harmonious digital landscape.