Understanding the Inner Workings: Variational Autoencoders Explained
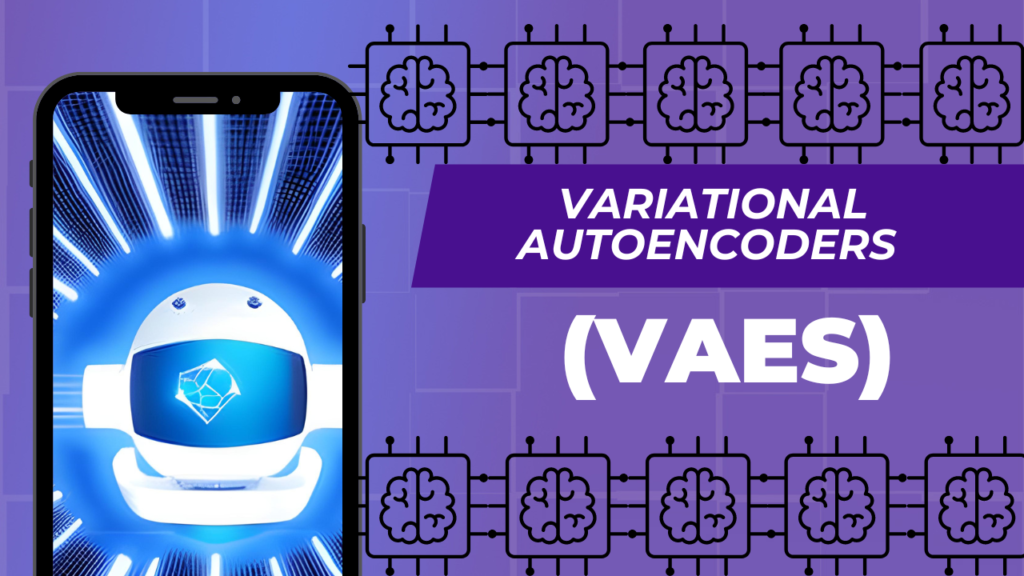
Variational Autoencoders (VAEs) represent a powerful fusion of probabilistic modeling and deep learning. At their core, VAEs are generative models that learn to encode and decode data in a latent space. This article provides an in-depth exploration of the inner workings of VAEs, elucidating how they balance the trade-off between data reconstruction and latent space regularization. By understanding the principles that govern VAEs, you’ll gain insights into how these models encode data’s essence and unleash their creative potential.
From Mathematics to Art: How Variational Autoencoders Foster Creative AI
The journey from mathematics to art takes an enchanting route through Variational Autoencoders (VAEs). These mathematical constructs find remarkable application in fostering creative AI endeavors. This article dives into how VAEs bridge the gap between data representation and creative expression. By mapping data to a latent space and reimagining it through the decoding process, VAEs empower machines to produce art, music, and even text that captures the essence of the original data in innovative ways.
Latent Space Exploration: Unveiling the Secrets of Variational Autoencoders
Central to the marvel of Variational Autoencoders (VAEs) is the latent space—a lower-dimensional representation of data. Latent space exploration is a journey into understanding how VAEs capture data’s intricate features within this space. This article delves into the mysteries of latent space, exploring how variations in the latent vectors translate into diverse data outputs. By unraveling the secrets of latent space, we gain insight into the transformative power of VAEs in generating novel, meaningful content.
Variational Autoencoders vs. Traditional Autoencoders: A Comparative Analysis
The battle of the encoders: Variational Autoencoders (VAEs) vs. Traditional Autoencoders. This article conducts a comprehensive comparative analysis, dissecting the strengths and weaknesses of each approach. While traditional autoencoders focus on accurate data reconstruction, VAEs introduce probabilistic modeling and latent space regularization. By understanding the differences and trade-offs, we gain insights into when to employ each technique based on the desired outcome—whether it’s precise data reconstruction or the generation of new and diverse content.
Generative Power Unleashed: Generating New Data with Variational Autoencoders
Variational Autoencoders (VAEs) stand as champions of generative power in the AI landscape. By encoding data into a latent space, manipulating latent vectors, and decoding them, VAEs unleash their ability to generate entirely new data samples. This article explores the generative prowess of VAEs, highlighting how they produce novel content while adhering to the learned data distribution. From art to music to text, the generative potential of VAEs opens doors to boundless creativity.
Anomaly Detection and Data Imputation: Applications of Variational Autoencoders
Variational Autoencoders (VAEs) excel beyond creativity—they’re adept at anomaly detection and data imputation. In situations where data is incomplete or corrupted, VAEs step in to reconstruct missing portions. This article delves into the applications of VAEs in anomaly detection and data imputation, discussing how their ability to learn underlying data distributions empowers them to identify outliers and seamlessly fill in gaps. By embracing these applications, industries can enhance data quality, decision-making, and overall operational efficiency.
Training Variational Autoencoders: Challenges and Best Practices
Training Variational Autoencoders (VAEs) presents unique challenges and requires careful consideration. From selecting appropriate loss functions to tuning hyperparameters, VAEs demand a nuanced approach. This article navigates the landscape of VAE training, offering insights into challenges like posterior collapse and strategies to mitigate them. By understanding best practices, practitioners can ensure that VAEs deliver on their promise of creative, data-driven generation.
Interpretable AI: Extracting Meaningful Insights from Variational Autoencoders
Interpreting the black box of AI is crucial, and Variational Autoencoders (VAEs) offer a pathway to interpretable AI. This article explores how the latent space of VAEs allows us to visualize and manipulate data representations, enabling meaningful insights. By decoding latent vectors and observing their effects, we gain a clearer understanding of the factors driving content generation. With interpretable AI, VAEs become not just generators of content, but also windows into data understanding.
Enhancing Transfer Learning: Variational Autoencoders in Multi-Domain Applications
Variational Autoencoders (VAEs) shine in multi-domain scenarios, enhancing transfer learning across diverse datasets. This article delves into how VAEs capture common features across domains, facilitating the transfer of knowledge. Whether for image synthesis, text generation, or data translation, VAEs enable seamless adaptation to new domains. By harnessing this power, practitioners can leverage pre-trained VAEs to excel in various applications, spanning domains and unlocking the potential of transfer learning.
Future Frontiers: Innovations and Trends in Variational Autoencoders
As AI evolves, the journey of Variational Autoencoders (VAEs) continues into exciting frontiers. This article peers into the future, exploring the innovations and trends that lie ahead. From advancements in training algorithms to novel applications in healthcare, from integrating VAEs with other AI techniques to ethical considerations, we uncover the trajectory that will shape the landscape of VAEs. By staying attuned to these future frontiers, we prepare to harness the full potential of VAEs in reshaping the creative and practical aspects of AI.
Conclusion: Navigating the Vast Horizons of Variational Autoencoders (VAEs)
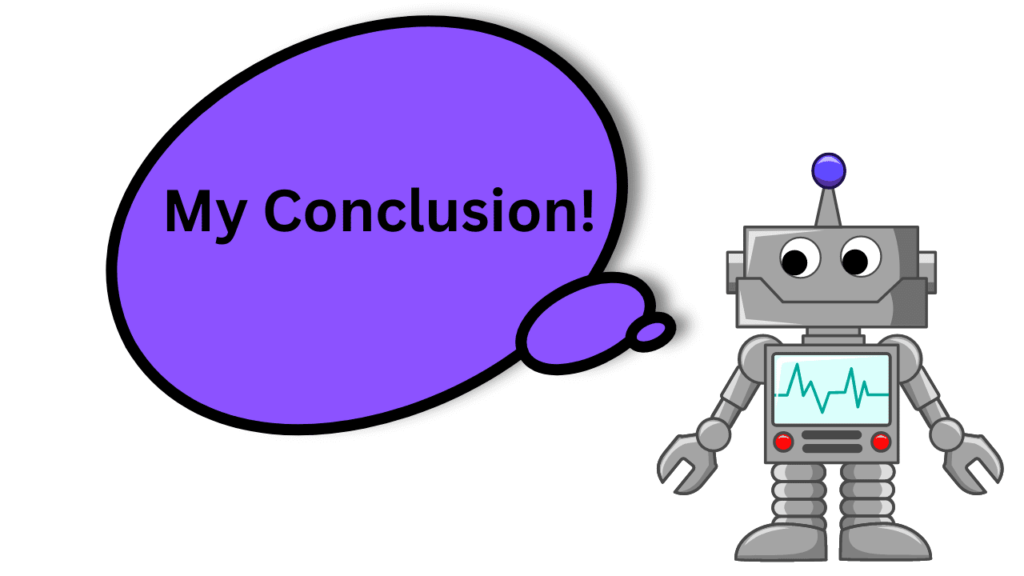
In the realm of AI and generative models, the journey through Variational Autoencoders (VAEs) unveils a world of creative potential, practical solutions, and limitless innovation. From decoding the inner workings of VAEs to harnessing their latent space for creative expression, we’ve seen how these models bridge the gap between mathematics and art. Latent space exploration has shed light on the mechanism that enables VAEs to generate diverse and meaningful outputs.
Comparing VAEs to traditional autoencoders, we understand the nuances that make each approach suitable for different scenarios. The generative power of VAEs is awe-inspiring, enabling machines to craft new data samples that span a spectrum of creativity. Beyond art, VAEs find their utility in anomaly detection, data imputation, and more—real-world applications that enhance data quality and decision-making.
Navigating the challenges and best practices of VAE training ensures that their creative power is harnessed effectively. Interpretable AI becomes a reality as VAEs unlock data insights, providing a window into the decision-making process. Multi-domain applications leverage VAEs’ versatility to enhance transfer learning and adaptation across various domains.
As we gaze toward the future, the frontiers of VAEs beckon with promise. From refining training algorithms to pioneering applications in cutting-edge fields, VAEs continue to push boundaries. By staying attuned to these trends, we position ourselves at the forefront of AI-driven creativity and innovation. With each iteration, each leap into the unknown, VAEs reaffirm their role as a transformative force shaping the future of artificial intelligence and beyond.